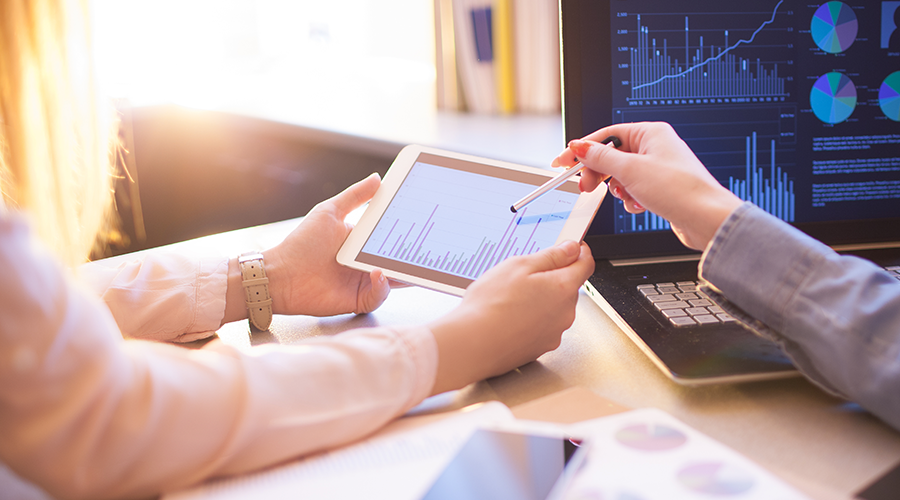
Self-service Analytics for Agile Business Decisions
Data is the new oil. Organizations use data analytics to uncover insights from their data and act on them to become more efficient, provide better products and services, and gain an overall competitive edge.
Every minute, tons of data bytes of all types are collected by organizations in the digitized world of today. Online or offline customer interactions, geo-location data, transactions within supply chains, orders and payments, workforce and HR operations, marketing campaigns – are all examples of internal data points that are collected.
When combined with other sources like consumer profiling and demographics, social media and market research, it becomes a veritable treasure of raw data that has the potential to be mined and distilled into real actionable insights using business analytics.
The Analytics Spectrum
Analytics uses data science to probe the collected records (aka Big Data) to answer pertinent business questions. Beyond diced and aggregated data tables, the story invoked from number crunching is represented by analysts using visuals – graphs and charts that make it easy to grasp the insights and make them highly impactful. It’s a field that is an amalgamation of both art and science.
In its most basic form, it is the combination, filtering, clustering and presentation of appropriate data to produce what may be termed Descriptive Analytics. This format helps in understanding what is happening with the business, highlights the key metrics and chalks out the trendlines. It is the first step towards data-driven decision-making for most organizations.
Descriptive analytics uses statistical modeling to represent historical data in a manner which makes it easy to comprehend and use while making strategic decisions.
Analysis and reporting on marketing campaign performance is an example of descriptive analytics. These types of visual reports can be built as needed from the historical data using self-service analytics tools.
Descriptive Analytics, however, leaves out any guidance as to the root cause of the trends being observed. With that added intelligence, we arrive at Diagnostic Analytics. It still focuses on historical patterns but delves deeper into the data to unearth insights as to why something happened. It is used to answer critical questions like “Why are online sales not growing?”, “What is causing manufacturing delays?” or “What are the opportunities to enhance customer service?”
Types of Analytics
Descriptive | Highlights key metrics to help understand what is happening with the business |
---|---|
Diagnostic | Focuses on historical patterns but delves deeper into the root causes |
Predictive | Extrapolates from past data trends to forecast the future |
Prescriptive | Performs real-time, multivariate analysis using ML to find patterns within data |
CXOs use diagnostic analysis to decide if and which organizational or process changes are required to bring about an improvement. However, they still need an estimate of the quantum of change that shall bring the desired outcome. They would like analytics to answer questions like “What will be the impact on gross revenue if the product price is reduced by 10%?”, “To meet demand spikes, is increasing finished goods inventory a better option than fulfilling upsurge with additional shifts?” or “What would the forecasted call volume be for customer X next week?” To answer these questions, analytics engines use statistical methods to extrapolate past data trends to forecast the future. This type of mature analytical framework is very useful as decision support inputs and is aptly called Predictive Analytics.
Machine Learning (ML) is a great addition to analytical engines as ML algorithms can be trained to find patterns within data, continuously improve with learning, and sift through a humongous amount of data blazing fast. This makes them a very potent addition to analytics tools and has been used by BI vendors to create Prescriptive Analytics. This type of analytics can perform multi-variate analysis in real-time and call out the best option. This is used in many industries like BFSI for fraud detection, deriving optimum discounts on travel booking sites, or matching the right agents to customer interactions in a call center.
Clearly, the value derived from the data analyses improves from descriptive to predictive analytical models. It also progressively becomes more difficult to implement, requiring increasing complexity in back-end systems, databases and analytical algorithms.
Critical Factors Driving Tangible Benefits From Analytics
In driving better decision-making, along with the right choice of analytics model to be used, two other factors are critically important, especially in today’s business environment:
Agility
Analysts at Gartner Data & Analytics Summit 2022 in Mumbai, India noted that “Data alone is unlikely to drive decisions. Design better decisions by improving the timing of decisions, accelerating decisions and connecting decisions.”
In an increasingly VUCA world, CXOs need to measure, analyze and adapt to ever-changing business environments at the speed of thought. Delayed decisions often prove to be damaging. As an example, when global supply chains were disrupted due to the Covid-19 pandemic, creating a sudden and unplanned uncertainty, companies that quickly adapted their vendors and logistics could maintain their delivery commitments.
Also, in a globally interconnected business world decisions made in silos, even though with agility, can be detrimental due to their ripple effects.
Analytics Process
The purpose of analytics is to answer a business question using data. During the early period of implementation, an IT or a specialized team of data analysts is usually tasked by CXOs for answering these questions. Data analysis is considered to be a highly specialized function involving technical know-how, involving programming and database management. Hence, the process remains IT-centric.
Even though this provides a great start in leveraging the data insights, a centralized analytics function soon appears to limit its scope. First, there can be a gap in understanding the business challenges of various functions by the analyst team. The skill set of the team is technical, which can make it challenging for them to appreciate departmental objectives. This leads to the analyst reports not addressing the real issues or not exactly answering the business users’ questions with actionable items, leaving businesses unable to apply the insights. Queries posed to a centralized analytics team may also take longer to answer, and hence, may not be useful for making timely decisions.
This could also be a result of the fact that the questions posed are unclear, or ambiguous. Sometimes, there is also a lack of clear appreciation among CXOs on what could be the scope of analytics and the data that they can leverage. For example, instead of asking questions like “How many active subscribers do we have?” a more perceptive use of analytics would be in asking “What would reduce the churn of active subscribers by 5% in 2023?”
Similarly, a question posed as “How can we increase cross-sell among our insurance products in the retail market by 3% y-o-y” and not “How many of our customers are buying more than one insurance product?” can deliver better results.
A Case for Self-Service Analytics
Even with the implementation of mature analytics models, the limitations posed by the process, dependency on the analytics team, their capability and the speed of execution create roadblocks in analytics becoming a very effective instrument in decision support.
Self-service analytics provides a very effective solution for bridging these critical gaps. Most BI vendors today provide self-service analysis features with simple-to-use, user-friendly tools.
When analytics queries are run by functional teams themselves, there is no disparity between the question asked, and the answer drawn from the data. Further, as departmental heads themselves sift through the data and charts, they are empowered to play around and delve deeper to uncover perceptions that may not have been obvious with their initial queries.
Self-service analytics also does away with the wait for the reports to be provided by the IT team, empowering CXOs and business users to analyze their data in real-time and make immediate decisions. The use of self-service analytics also frees up IT/ analytics resources for developing better data models and algorithms, further improving the value drawn from the data.
Challenges in Implementing Self-Service analytics
While eliminating several issues discussed earlier, implementation and use of self-service analytics have their own sets of challenges. Some of them are:
Data Integration and Readiness: Organizational databases range from legacy, on-prem to cloud-native ones. At the same time, worksheets continue to be used extensively. Also, the variety of data captured ranges from transactional, structured, unstructured, audio/video, pictorial or geophysical. The toughest challenge is to integrate and prepare these databases and data types so that they can be subjected to self-service analytics, producing results in real-time. Thankfully, there are several database or independent vendors who have ready solutions for data readiness. Solutions are also built into some BI platforms, like Intellicus.
Data Literacy: Self-service analytics can be best used by those who have competencies of working with data, are curious, have analytical abilities and are able to ask the right questions to derive information from it. CXOs should assess the level of competency within their teams, and work on developing them as a prerequisite to building a culture of using data-driven decision-making.
Data Security and Governance: Implementation of self-service analytics requires an opening up of access to organizational data for all users. This demands a standardized approach to data governance that outlines data ownership, privacy, confidentiality, and security while controlling access according to need. Checks are required to be put in place to restrict access (or anonymize) of sensitive data like payroll, customer profiles, etc.
Explore the Power of Self-Service Analytics with Intellicus
Self-service analytics tools can be used across the analytics spectrum discussed earlier. It can have a broad range of features that may be used for calling out descriptive analytics, or forecasting as in predictive analytics, or even automating the analytics output to drive recommendation engines or optimal discounts. The self-service analytics interface of Intellicus enables business users to run queries, generate reports and drill down into data to unearth insights without having to lean on their IT team.
Descriptive analytics pulled using Intellicus’s BI and Analytics engine from global sales performance data.
The tools menu has an intuitive interface with drop-downs, checkboxes, or data range selections. Complex data manipulation and query functions are automated and driven by algorithms. Statistical operations, filters and summarization features are pre-built into the tool. It also has extensive data visualization options for easy comprehension, with click-and-point drill-down capabilities to explore the data.
Conclusion
Various factors play a role in driving benefits out of a company’s investment in Analytics. The first step is implementing the right analytical models to be used at various decision points. that would result in gaining a competitive edge. This can range from Descriptive, Diagnostic, Predictive, Prescriptive, or a combination based upon the type of organization’s data and systems and processes.
While designing analytics usage, the timeliness of decision support inputs and their pointedness are critical factors. Self-service analytics serve as a tool that empowers users to leverage the data to find answers to their critical business questions by themselves, with agility, and without the need for complex coding skills.
With self-service analytics, CXOs and teams gain an overall maturity and thrive in a data-driven culture using decision intelligence securing a competitive edge for the organization.