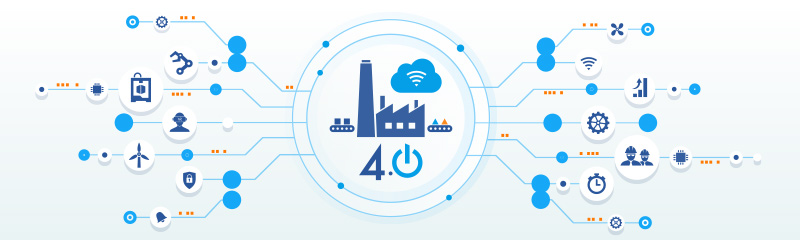
Predictive Analytics in Manufacturing: Keeping pace with Industry 4.0
The market across the world is transforming at a tremendous pace. Customer expectations, competition, innovations are becoming the focal point for companies in terms of manufacturing. These changes have led to modern supply chains, where the operators need to always be a step ahead of the process, to be profitable. This certainly cannot be achieved without utilizing predictive analytics. On a deeper level, it not just enhances the complete process but also makes sure, no opportunities get missed. This need has made the industry seriously consider and utilize predictive analytics in their everyday operations.
Previously, to know the production output, machine efficiency, or identify an error, long and time taking manual processes were followed. Even, some traditional predictive practices also existed, which were not as effective. However, this scenario completely changed with the arrival of IIoT and when all the right data started getting captured and analyzed resulting in accurate predictive insights. Predictive manufacturing analytics coupled with IIoT has empowered manufacturers to optimally use data coming from SCADA, sensors, and from other sources, leading to the path for better processes and ROI. Let us have a closer look at how predictive analytics in manufacturing is transforming various facets of the industry:
- Manufacturing a product can be a long and complicated process. From sourcing raw material, managing machinery to understanding fluctuating demand, manufacturers have to deal with multiple components before even starting the production. The raw material cost can be directly impacted by demand, availability, shipping, and more and is a major contributing factor in the final product cost. Along with this, any hampering in the production process or machinery directly impacts the result. The general manufacturing execution systems are unable to create a 360 predictive view of the process which an AI-enabled MES can do. Right from demand forecasting, foreseeing the raw material delivery, predicting machine maintenance or downtime to enhancing operational efficiency and output quality, the ML-driven MES system can save cost and time, prevent revenue leakages and improve ROI by leaps and bounds.
- Manufacturing equipment undergoes extreme conditions like high temperatures, vivid motions, high pressures, and more on an everyday basis. This can cause a lot of wear and tear in the machines and the components and can lead to breakdowns which can be an overall expensive business for the manufacturers including machine and workforce efficiency issues, lost production, and quality issues. With predictive analytics, machines, their components, and other parameters can be monitored and tracked in real-time by aggregating data from sensors and any potential future breakdown can be predicted. It can also provide much deeper level insights like these:
- Demand forecast is one of the most essential use cases of predictive analytics. It enables manufacturers to efficiently provide customers what they ask for at the right time. IoT and predictive analytics enable manufacturers to gather the right data and identify trends, patterns, and existing gaps to better understand the manufacturing process. Predictive analytics essentially combines traditional demand forecasting with risk management. It can consider multiple factors like raw material availability, consumer behavior, sales data, weather conditions, supplier and shipping issues, and other factors to accurately forecast demand. Not just this, it also enables identify unknown factors that affect demand and their level of correlation. This also enables better inventory management by preventing overproduction and dead stocks. Manufacturers can now only make the right predicted number of products to satisfy consumer needs, maximize revenue, avoid any loss due to products being out of stock, and save valuable time and resources.
- Workforce management in the manufacturing industry isn’t easy and has many issues. From hiring skilled labor to maintaining their productivity, the HR management face hurdles of competition in the recruitment and understanding possible productivity components. With proficient use of advanced analytics, the HRs can get detailed insights regarding hiring, training, and workforce efficiency. By predicting consumer demand and potential machine failure, the HRs can define the staffing needs beforehand, they can understand which factors are affecting employee productivity and engagement like shifts, machine efficiency, breakdowns, safety incidents, and more. The current market recruitment trends and salary benchmarks can be understood. HRs can also further identify essential KPIs to track for enhanced overall operations. With predictive analytics, employers can also see red flags and know which employees are at risk of attrition, and take appropriate actions.
- Predictive analytics can also prove to be instrumental in employee safety and workplace health. Operators can predict potential incidents and take proactive actions to avoid them ensuring a safe environment for workers and improve productivity and overall morale in the workforce.
- One of the most vital applications of predictive analytics in manufacturing is maintaining the quality of both products and outputs. It can identify any upcoming quality problem in time to avoid any loss on production and expensive product callbacks. This enhanced quality consequently minimizes warranty claims, inspection work, and scrap work. This quality analytics help in managing machine maintenance schedules and enabling proactive actions. Ultimately, it elevates the customer experience and enhances brand equity over time.
- Another use of predictive analytics is in making the machines and production more efficient with minimum waste. Manufacturers can monitor the machine performance in real-time and the data generated by sensors and devices can be analyzed to understand the utilization efficiency and pattern. This can help in taking preventive actions like managing machine schedules by shutting them off or switching them on. The data analysis can inform the manufacturers if the machines are being utilized at full efficiency and what actions could be taken to maximize it.