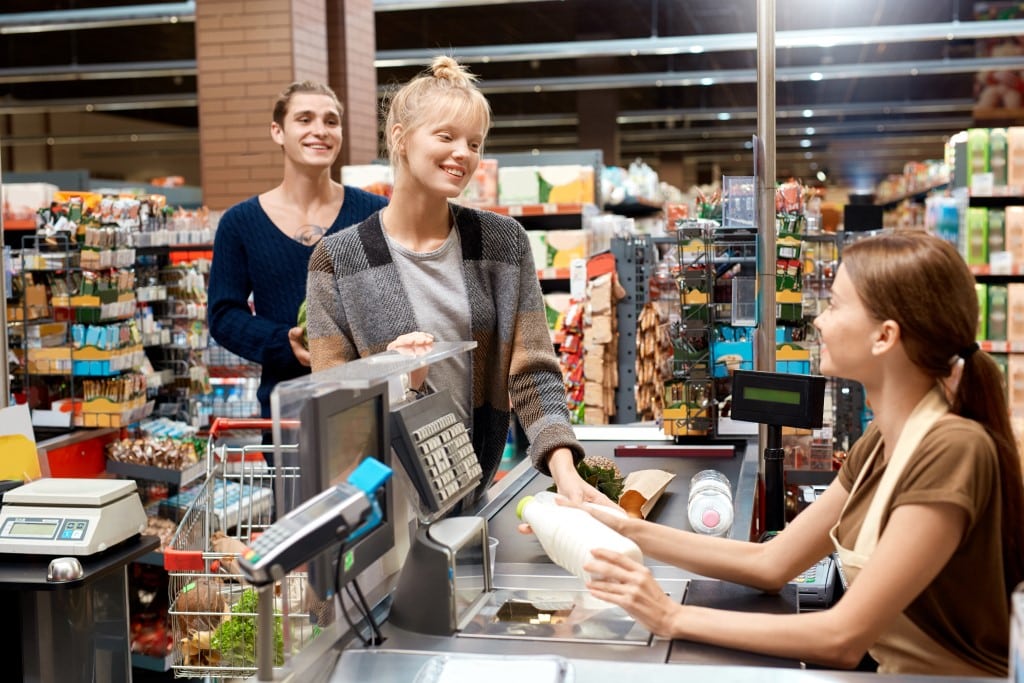
How Customer Analytics Leads to Better Business Decisions
Customer service and support are two mission-critical factors that every organization needs to focus on. For a more connected, informed and empowered customer base, many enterprises today are predisposed toward understanding customer behavior using analytics. A 2023 Gartner Survey found that 84% of customer support decision-makers cited customer data analytics as crucial for achieving organizational goals.
Benefits of Customer Analytics
Customer analytics provides valuable information at several touchpoints in the purchase journey of customers. Companies can fill gaps based on this data and change course, leading to increased conversion rates and sales. For example, a payment gateway mobile app used an analytics platform to learn that customers were unable to download the app due to incompatible operating systems. Once this problem was fixed, the company experienced instant sales conversions.
Similarly, analytics tools help identify which sales channels are working and which are not. This empowers businesses to redirect their marketing efforts and save on customer acquisition costs. Monitoring customer data helps companies validate their product updates and features. They can personalize the user experience by identifying pain points in the customer journey and resolving those issues quickly. Moreover, prescriptive tools help forecast future behaviors, enabling businesses to meet customers where they are.
Customer Analytics and Buyer Journey
Every customer goes through three stages of decision-making before buying a product, namely, awareness, consideration, and decision. This is called a buyer’s journey. With actionable data across all channels, organizations can improve customer experiences at every stage of this process – from awareness and purchase to post-purchase and advocacy.
Here’s how different types of analytics help gather insights at each step of the way:
Descriptive Analytics
Descriptive analytics refers to the statistical interpretation that helps analyze historical customer data, finding meaning within it. When a customer is at the ‘Awareness’ stage of the purchase journey, this type of analytics describes an outcome or phenomenon and identifies patterns and relationships. In short, this analysis answers the question: What happened?
For example, an eCommerce company analyses sales data to see if the purchase probability increases due to any one factor, which may be ‘location’ in this case. Here, descriptive analytics comes into play when they compare these sales for several years using the same parameters and identify any pattern—if the sales shot up every year in a particular location or did it happen just once this year. Depending on insights drawn, they can create a clearer path of action focused on capitalizing the location garnering highest sales.
Diagnostic Analytics
With diagnostic analytics, businesses can answer: Why did it happen? In the context of data analytics, this type involves finding out the reasons for certain outcomes with behavioral analysis of the customers, such as their engagement patterns, actions and preferences.
For example, a fashion apparel brand may run diagnostic analytics through anonymous surveys to understand if its products resonate with a particular customer demographic. Through an analytics solution, the data may present a case for allocating more resources to make the target demographic comfortable with the brand.
Predictive Analytics
What might happen in the future? Predictive customer analytics answers this question. It uses historical data on the purchases and shopping carts to create predictive models that can forecast future customer behaviors, such as the types of customers likely to buy the product, which kinds of customers will churn or how sales might trend. This involves using statistical modeling and machine learning methods to analyze customer data at the ‘purchase’ and ‘post-purchase’ stages of the customer journey.
For example, the Oakland Aesthetics baseball team used a predictive analytics tool to assemble a competitive team by analyzing evidence-based data to predict game outcomes, taking them to two consecutive playoffs.
Prescriptive Analytics
Finally, prescriptive customer analytics answers: What should be done next? Known as ‘the future of data analytics’, this type of analytics uses customer information to determine an optimal course of action. Organizations use machine-learning algorithms to process large amounts of data more efficiently.
For example, if a dataset says that 50% of customers were ‘unsatisfied’ with a business’ customer service team, the algorithm may suggest additional training for the staff.
How Does Customer Analytics Work?
Customers want convenience, speed and personalization when investing in a new product or service. In a 2022 Statista survey, 94% of the customers said a positive experience made them buy from that business again, while 84% recommended a business offering excellent customer experience. As such, organizations that persevere to improve their customer service levels will always stay ahead of the curve.
Customer analytics provides the context and the reference for enhancing and personalizing business offerings. It steers organizations towards making changes, improving processes and implementing insights.
The following is the step-by-step process they can follow for customer analytics:
#1 Defining and Setting Customer Success Goals
An organization needs to determine its customers’ pain points, its own unique selling propositions to solve those pain points, what kind of factors are leading to customer unhappiness and which factors make customers come back to the said organization’s solution. These considerations, along with business goals, determine customer success and work like yardsticks for implementing a customer data analytics tool.
#2 Data Collection
Once customer success is defined, the next step is to collect data from various touchpoints, such as customer surveys, transactional histories, customer feedback, social media channels, customer service centers, transaction channels (offline and online), etc. One way to simplify this process is to use a high-performance platform that consolidates customer profiles across various data sources and enables easy structure, management and interaction with customer data.
#3 Data Segmentation
Data segmentation involves dividing customer data into small segments, like geography, demographics and behaviors, such as purchasing patterns, lifestyle habits, interests and beliefs, etc.
#4 Data Visualization
As the name suggests, the next step in the process is creating charts, graphs and dashboards to better understand the collected data. Visualization makes it easier to comprehend information, identify relationships and patterns, recognize correlated parameters and spot trends.
#5 Data Analysis
This step involves customer data mining and behavior modeling to gauge and predict users’ responses to marketing and brand campaigns. BI tools help analyze customer behavior across a sales funnel to identify gaps and bottlenecks.
Users can extract meaningful information from channels like call center logs, customer social media interactions, sales emails, feedback, etc., and identify areas for improvement. Data analysis also involves customer satisfaction analysis, which measures customer satisfaction ratings or service experiences. Other types of customer data analytics include customer churn analysis, customer sentiment analysis, customer life span prediction, etc.
Final Thoughts
Customer analytics usually comes in the form of software that decodes the actions of the consumers for deeper understanding. When consumer behavior is tracked and analyzed at every stage of the buyer’s journey, companies find it easier to break big challenges, such as changing customer expectations, understanding customer needs or customer retention, into manageable solutions.
The best software allows businesses to segment customers and personalize experiences while predicting and preventing churn. Insights, such as purchase history or consumer behavior over a specific period, positively impact average transaction value by filtering opportunities to upsell or cross-sell to the existing customer base. In short, customer analytics is the gateway to enhanced customer experience and growth.