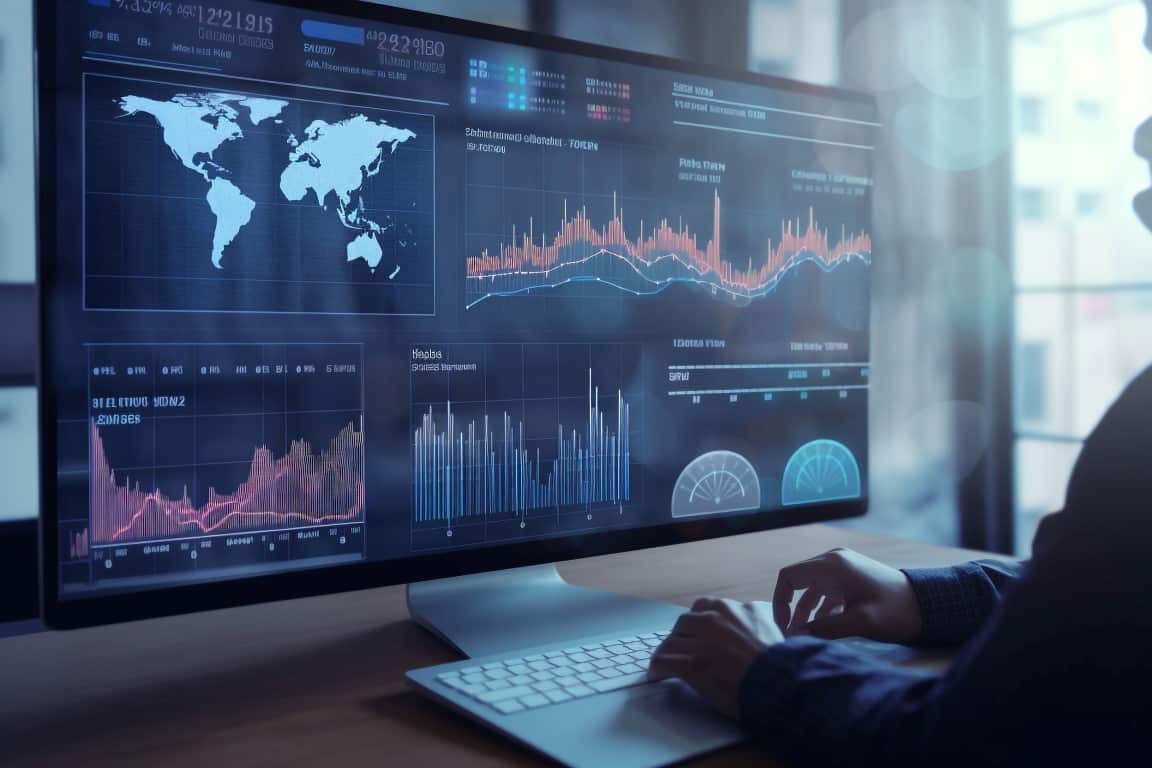
Business Intelligence: Benefits, Trends and Future Scope
In the ever-evolving landscape of modern businesses, the ability to make informed decisions has become a linchpin for success. In this dynamic environment, organizations turn to Business Intelligence (BI) as a powerful ally in navigating the complexities of data analysis and decision-making.
At its essence, Business Intelligence refers to the strategies and technologies employed by enterprises to collect, analyze and manage business information. The primary goal is to enhance the quality and timeliness of data available for decision-making processes. In a world saturated with data, BI acts as a beacon, illuminating the path to actionable insights and strategic planning.
Business Intelligence is a technology-driven process that involves a series of steps that collectively enable organizations to transform raw data into meaningful and actionable insights.
Data Collection: Creating The Foundation of BI
At the heart of Business Intelligence lies data, the lifeblood of informed decision-making. The starting step in the BI process is data collection. Organizations gather data from various sources, both internal and external, to create a comprehensive dataset. Internal sources may include customer relationship management (CRM) systems, enterprise resource planning (ERP) systems and transactional databases. External sources could range from market research reports to social media data.
Data collection is not merely a one-time process but an ongoing one. Continuous updates ensure that the information used for analysis remains relevant and reflects the current state of the business environment. The quality and accuracy of the data collected directly shape the effectiveness of BI outcomes.
Data Preparation: Shaping Raw Data into Actionable Insights
Raw data, as collected from diverse sources, is often unstructured and incoherent. The next step in the BI process is data preparation, where this raw data is processed, cleaned and transformed into a usable format. This stage involves cleaning up inconsistencies, handling missing values and structuring the data in a way that facilitates analysis.
Data preparation also involves storing the processed data in data warehouses or in the cloud. This ensures accessibility, security and scalability, allowing organizations to handle vast amounts of data efficiently.
Data Analysis: Unveiling Insights Through Methodologies
Once the data is collected and prepared, the core of BI comes into play – data analysis. This phase involves employing various methodologies to extract meaningful insights from the dataset. Some of the key analytical techniques include:
Data Mining: Identifying patterns and relationships within large datasets to discover hidden insights.
Process Analysis: Evaluating the efficiency of business processes to identify bottlenecks and areas for improvement.
Performance Benchmarking: Comparing the organization’s performance against industry standards to gauge competitiveness.
Data analysis is not a one-size-fits-all approach; rather, it is tailored to the specific needs and goals of the organization. The insights gained during this phase lay the groundwork for informed decision-making.
Data Visualization: Translating Complex Data into Understandable Formats
While raw data and analytical results provide valuable insights, they might be challenging for non-technical stakeholders to comprehend. Data visualization addresses this challenge by presenting complex data in visually appealing and easily digestible formats. BI tools create visual representations such as dashboards, charts and graphs, offering a quick overview of critical metrics.
Effective data visualization is more than just aesthetic appeal; it enhances the interpretability of data, enabling decision-makers to grasp trends, patterns and outliers intuitively. This visual layer bridges the gap between raw data and actionable insights, fostering a more comprehensive understanding of the business landscape.
Decision-Making: Empowering Business Users
Armed with the analyzed and visually presented data, business users can make informed decisions aligned with organizational objectives. The goal of BI is to provide the tools and insights necessary for strategic and operational choices to decision-makers at all levels of an organization.
BI tools often provide interactive features, allowing users to drill down into specific data points, conduct ad-hoc analysis and explore data on their own. This self-service aspect reduces dependency on IT departments for generating reports and promotes a culture of data-driven decisions throughout the organization.
Reporting and Dashboard: BI platforms facilitate the creation of interactive and dynamic reports and dashboards. These tools enable users to present key performance indicators (KPIs) and other critical metrics in a visually compelling manner.
Self-service Analytics: Empowering business users to conduct their analyses is a key feature of modern BI platforms. Self-service analytics allows users to create reports and perform analyses without requiring the intervention of IT departments, fostering a culture of data-driven decisions at every level of the enterprise.
Collaboration and Sharing: BI platforms often include features that enable collaboration among team members. Users can share reports, dashboards and insights, nurturing a collaborative approach to decision-making.
Mobile Accessibility: In today’s dynamic business environment, the ability to access BI tools on mobile devices is crucial. BI platforms typically offer mobile applications or responsive design for on-the-go access to critical business insights.
Security and Governance: Maintaining the security and integrity of data is a top priority for organizations. BI platforms include robust security features and governance mechanisms to ensure that sensitive information is protected and compliance requirements are met.
The Emergence of Business Intelligence
The origins of BI can be linked to the 1960s when corporations began to realize the untapped potential of their data. During this era, the business landscape was witnessing a shift towards computerization. The first wave of BI was characterized by the development of Decision Support Systems (DSS), which aimed to assist managers in making informed decisions.
1960s-1970s: The Rise of Decision Support Systems
Decision Support Systems marked the embryonic stage of BI, focusing on providing executives with tools to analyze internal data. These early systems allowed managers to access and manipulate data to drive actionable insights. However, the accessibility and usability of these systems was limited, as they often required specialized skills to operate.
1980s-1990s: The Advent of Executive Information Systems (EIS)
As technology advanced, the 1980s saw the emergence of Executive Information Systems (EIS). These systems were designed to cater specifically to top-level executives, offering a more user-friendly interface compared to their predecessors. EIS brought a graphical representation of data to the forefront, allowing executives to visualize key metrics and trends easily.
The 1990s witnessed the proliferation of relational databases, enabling more robust data storage and retrieval. This technological leap laid the groundwork for the evolution of BI by providing a structured environment for data analysis.
Late 1990s-2000s: The Internet and Data Warehousing
The late 1990s and early 2000s were marked by the advent of the internet and the integration of data warehousing into the BI landscape. Data warehousing allowed organizations to consolidate data from various sources into a single repository, facilitating more comprehensive analysis.
The internet played a pivotal role in democratizing access to data. With the rise of web-based BI tools, businesses could now provide access to insights beyond the confines of the executive suite. This shift towards greater accessibility marked a turning point, emphasizing the need for a broader, more inclusive approach to BI.
Early 2000s-2010s: The Rise of Business Intelligence Platforms
The early 2000s witnessed the emergence of comprehensive Business Intelligence Platforms (BIP). These platforms integrated various BI tools, offering a more cohesive solution for organizations. The focus shifted from isolated tools to integrated suites that encompassed reporting, analytics and data visualization.
Key players in the software industry, such as Microsoft, IBM and SAP, began to dominate the BI landscape, providing end-to-end solutions that catered to the diverse needs of businesses. This era also saw the rise of self-service BI, empowering users across different departments to create and explore reports without the need for specialized IT support.
2010s-Prеsеnt: The Era of Big Data and Advanced Analytics
As we entered the 2010s, the business landscape underwent a seismic shift with the explosion of Big Data. Traditional BI tools faced challenges in handling the sheer volume, velocity and variety of data generated by modern enterprises. This led to the evolution of BI tools to incorporate advanced analytics, machine learning and predictive modeling.
The concept of data discovery gained prominence, allowing users to explore data sets intuitively. Visualization tools became more sophisticated, enabling the creation of interactive dashboards that could convey complex insights in a digestible format.
The Current Landscape: BI in the Age of Digital Transformation
In the present day, Business Intelligence has become an integral part of digital transformation strategies. Organizations are leveraging BI to not only gain insights into historical data but also to predict future trends and prescribe actions for optimal outcomes.
Cloud-based BI solutions have gained popularity, providing scalability and flexibility to meet the dynamic needs of businesses. The integration of artificial intelligence and machine learning has further enhanced BI capabilities, enabling automated insights and predictive analytics.
The Future: Augmented Analytics and Beyond
Looking ahead, the future of Business Intelligence is poised for exciting advancements. Augmented analytics, which leverages AI and machine learning to automate data preparation, insight discovery and sharing, is expected to play a crucial role. Natural language processing (NLP) is also making BI more accessible, allowing users to interact with data using everyday language.
Additionally, the Internet of Things (IoT) will contribute to the growth of BI by providing a wealth of real-time data from interconnected devices. This influx of data will necessitate more advanced analytics capabilities to derive meaningful insights.
Implementing BI tools has a multitude of benefits for any organization seeking to take advantage of the vast amounts of data available to them. By formatting and analyzing data from various sources, the insights that are derived can help businesses to achieve:
Data-Driven Decision Making
By leveraging BI tools, organizations can move beyond intuition and gut feelings, relying on concrete data and analytics to guide their strategies. This shift ensures that decisions are not based on assumptions but on a comprehensive understanding of the current business landscape.
Improved Operational Efficiency
BI tools empower organizations to streamline their operations by providing a clear view of their processes. Through analytics and reporting functionalities, businesses can identify inefficiencies, bottlenecks or redundancies in their workflows. This, in turn, allows for targeted improvements and optimizing processes, ultimately leading to increased operational efficiency.
Enhanced Visibility
BI tools provide a panoramic view of an organization’s data, offering insights into various facets of the business. This visibility is crucial for understanding customer behavior, market trends and internal operations. With a comprehensive understanding of these factors, businesses can make strategic decisions that align with their objectives.
Better Resource Utilization
Informed decision-making extends to resource allocation as well. BI helps organizations identify where resources are most needed and where they may be underutilized. This optimization ensures that time, money and manpower are directed towards areas that will yield the highest returns, fostering a culture of efficiency and productivity.
Real-Time Reporting and Analysis
Traditional reporting methods often involve time-consuming processes, leading to delays in obtaining critical information. BI tools, on the other hand, enable real-time reporting and analysis. This promptness is invaluable, especially in fast-paced industries where being a step ahead can make a significant difference.
Competitive Advantage
In a world where every edge matters, BI provides a substantial competitive advantage. Businesses can stay ahead of the market by examining market trends, customer behavior, and competitor actions. By making strategic decisions based on data-driven insights, organizations position themselves to not only survive but thrive in competitive markets.
Enhanced Customer Experience
Understanding customer needs and preferences is essential for delivering a personalized and satisfying experience. BI tools can analyze customer data, including purchase history, feedback and interactions, to create a comprehensive profile. This profile assists businesses to tailor their products or services, anticipate customer needs and provide a seamless and delightful customer experience.
Increased Revenue Opportunity
The insights gained through BI can directly impact revenue generation. By identifying high-performing products, target demographics and effective marketing strategies, businesses can focus their efforts on areas that drive revenue growth. Additionally, BI tools can help in pricing optimization, ensuring that products are competitively priced based on market demand and competitive benchmarks.
Risk Management
In an era of constant change and uncertainty, effective risk management is crucial. BI tools provide predictive analytics and scenario modeling capabilities, allowing organizations to assess potential risks and develop strategies to mitigate them. This proactive approach minimizes the impact of unforeseen challenges, safeguarding the stability and continuity of the business.
Scalability
As businesses grow, so does the volume of data they generate. BI tools are designed to scale alongside an organization’s needs. Whether it is a startup or an established enterprise, BI solutions can adapt to handle increasing data complexity and ensure that analytical capabilities remain robust.
Compliance and Governance
In industries where compliance with regulations is paramount, BI tools play a vital role in ensuring adherence. They provide features for data governance, audit trails and security measures, helping organizations maintain compliance with industry standards and legal requirements. This not only mitigates risks but also builds trust with stakeholders.
Democratization of Data
BI tools empower users at all levels of an organization to access and analyze data. This democratization of data reduces dependency on IT departments for generating reports and insights. Business users can explore data, create visualizations and derive insights independently, fostering a culture of self-service analytics.
Adaptability to Change
The business landscape is in a constant state of flux, with market trends, customer preferences and other external factors evolving rapidly. BI tools enable organizations to adapt to these changes by providing real-time insights. Whether it’s adjusting marketing strategies, optimizing supply chains or pivoting business models, BI facilitates agility in decision-making.
Measurable ROI
Implementing BI is an investment, and like any investment, organizations seek a return. BI tools provide a measurable return on investment (ROI) by contributing directly to growth, cost savings and overall operational improvements. Tracking key performance indicators (KPIs) allows businesses to assess the impact of BI on their bottom line.
Examples of Successful BI Implementations
As the adoption of BI has seen a substantial rise, there are a plethora of organizations across various industries, from е-commerce giants personalizing user experiences to healthcare organizations optimizing patient care, where BI has become a catalyst for transformative change.
Amazon – Leveraging BI for Customer Personalization
Amazon, the е-commerce giant, is a prime example of how BI can be used to enhance customer experiences. Amazon employs sophisticated BI algorithms to analyze customer behavior, preferences and purchasing history. By leveraging this data, they provide personalized product recommendations to each user, creating a tailored and engaging shopping experience.
Amazon’s BI system doesn’t stop at recommendations; it also optimizes inventory management, pricing strategies and supply chain operations. Through real-time analytics, Amazon can adjust prices dynamically based on factors such as demand, competitor pricing and inventory levels, ensuring competitiveness and maximizing profitability.
Netflix – Data-Driven Content Recommendations
Netflix, the streaming service that has revolutionized the entertainment industry, relies heavily on BI to keep viewers engaged. The platform analyzes user interactions, viewing habits and ratings to offer personalized content recommendations. The recommendation engine uses machine learning (ML) algorithms to predict what users might enjoy based on their historical data.
Additionally, Netflix uses BI to make strategic content decisions. By analyzing viewer demographics, preferences and regional trends, Netflix produces original content that resonates with its audience. This data-driven approach not only enhances user satisfaction but also contributes to the success of Netflix’s original productions.
Starbucks – Brewing Success with BI Analytics
Starbucks, the global coffeehouse chain, uses BI to optimize its operations and enhance the customer experience. Through the analysis of sales data, customer preferences and foot traffic, Starbucks can make informed decisions about store locations, inventory management and product offerings.
Starbucks’ BI system aids in real-time adjustments to product pricing and promotions. For example, during certain seasons or times of the day, Starbucks may adjust prices or launch targeted promotions to drive sales. This data-driven approach not only improves profitability but also ensures that customers receive relevant and timely offers.
Google – BI for Enhanced User Experience
Google, synonymous with internet search, relies on BI to continually refine and improve its search algorithms. Google Analytics, a widely used BI tool, provides insights into user behavior, website traffic and the effectiveness of online advertising. By analyzing this data, Google can enhance the relevance and accuracy of search results, delivering a better user experience.
Beyond search, the Google Cloud Platform offers a suite of BI tools that businesses can leverage to analyze and visualize their data. This enables organizations to make data-driven decisions, optimize processes and uncover new opportunities for growth.
Tеsla – BI in Manufacturing and Supply Chain
Tesla, the innovative electric vehicle manufacturer, employs BI to streamline its manufacturing processes and supply chain management. Through the use of BI and analytics, Tеsla can monitor and optimize production workflows, identify bottlenecks and ensure the efficient utilization of resources.
BI tools help Tesla manage its complex supply chain by providing real-time visibility into inventory levels, supplier performance and demand forecasts. This enables proactive decisions, reducing the risk of disruptions and ensuring a smooth flow of materials to support production.
Healthcare Industry – BI for Patient Care and Operational Efficiency
In the healthcare sector, BI plays a crucial role in improving patient care and operational efficiency. Electronic Health Record (EHR) systems, integrated with BI tools, allow healthcare providers to analyze patient data, track treatment outcomes and identify patterns that can inform medical decisions.
Hospitals and healthcare organizations use BI to optimize resource allocation, reduce costs and enhance overall operational efficiency. For example, BI can help predict patient admission rates, allowing hospitals to allocate staff and resources accordingly.
Financial Institutions – BI for Risk Management and Fraud Detection
Financial institutions leverage BI to manage risk, detect fraud and enhance customer satisfaction. BI tools analyze vast amounts of financial data in real-time to identify anomalies, patterns and potential risks. This proactive approach enables financial institutions to take swift action to prevent fraudulent activities and protect their customers.
Moreover, BI supports strategic decisions in areas such as investment management, loan approvals and customer relationship management. By analyzing market trends and customer behavior, financial institutions can make informed decisions that align with their business objectives.
Crafting a Business Intelligence Strategy
A BI strategy provides a roadmap for organizations to collect, analyze and leverage data to make informed decisions. Following are the key steps and considerations involved in creating a robust BI strategy that aligns with your business objectives.
Define Business Objectives and KPIs:
The first step in creating a BI strategy is aligning it with business objectives. Clear articulation of what the organization aims to achieve through BI is necessary. Whether it is to increase sales, improve customer satisfaction or optimize internal processes, the requirements must be clearly ascertained. Once the objectives are defined, identification of the Key Performance Indicators (KPIs) that will measure success is vital.
For example, if the goal is to enhance customer satisfaction, relevant KPIs might include customer purchase scores, response times and resolution rates.
Assessing the Current State and Identify Data Sources:
Conducting a thorough assessment of the organization’s current state concerning data is required. Identifying existing data sources, both internal and external, is an essential step. This could include data from customer relationship management (CRM) systems, enterprise resource planning (ERP) systems, spreadsheets and external market data.
Evaluating the quality and reliability of the data sources is also paramount. Inconsistent or inaccurate data can undermine the effectiveness of a BI strategy. Data cleansing and validation processes may be necessary to ensure the integrity of the information.
Choosing the Right BI Tools:
Selecting the right BI tools is a critical decision in the strategy creation process. Consideration of factors such as the complexity of data, the technical expertise of the team and the specific requirements of the business must be kept in mind.
Ensuring that the chosen tools align with the organization’s technology stack and can seamlessly integrate with existing systems is imperative. Scalability is also a crucial consideration, especially if an organization plans to expand its data capabilities in the future.
Developing a Data Governance Framework:
Establishing a robust data governance framework is essential for maintaining data quality, security and compliance. Clearly defining roles and responsibilities for data management is important to ensure that data is treated as a valuable organizational asset. Implementing access controls to restrict data access based on roles and regularly auditing and monitoring data usage goes a long way for sustained success of the strategy. A well-defined data governance framework instills confidence in the reliability of data, fostering trust among decision-makers.
Creating a Data Architecture and Infrastructure Plan:
Defining the architecture and infrastructure required to support a BI strategy is key. Additionally, determining whether an on-premises, cloud-based or hybrid solution is most suitable for an organization must be done. Factors such as data storage, processing power and scalability must be taken into consideration. Cloud-based solutions offer flexibility and scalability, allowing organizations to adapt to changing data requirements.
Establishing Data Integration and ETL Processes:
Efficient data integration and Extract, Transform, Load (ETL) processes are crucial for ensuring a seamless flow of data from various sources to the BI platform. The data integration tools and ETL processes that align with the organization’s needs must be identified. Automation of ETL processes can significantly reduce the time and effort required to prepare and transform data for analysis.
Implementing Data Security Measures:
Security should be a top priority in any BI strategy. Measures to protect sensitive data, both in transit and at rest, need to be implemented. This includes encryption, access controls and regular security audits.
Additionally, the team needs to be educated on data security best practices to ensure that everyone understands their role in maintaining a secure data environment.
Designing User-Friendly Dashboards and Reports:
The ultimate goal of a BI strategy is to empower decision-makers with actionable insights. Intuitive and user-friendly dashboards and reports that cater to the needs of different stakeholders must be designed. The audience and their level of technical expertise needs to be considered when designing visualizations.
Providing Training and Support:
Even the most advanced BI tools are only as effective as the users leveraging them. Comprehensive training must be provided to ensure that the team has the skills and knowledge to use the BI tools effectively. Offering ongoing support and resources to address any questions or challenges that may arise is required.
Monitoring and Iteration:
The implementation of a BI strategy is not a one-time process but an ongoing one. Regularly monitoring the performance of the BI system and gathering feedback from users is needed. The use of key metrics and KPIs to assess the impact of the BI tool on an organization’s objectives is a must.
Refining the BI strategy based on the insights gained and changes in business requirements is essential to embrace a culture of continuous improvement and stay ahead in the dynamic business landscape.
Creating a Business Intelligence strategy is a multifaceted process that involves aligning technology, people and processes with organizational goals. By defining objectives, assessing data sources, choosing the right tools and establishing robust governance and security measures, organizations can craft a future-ready BI strategy that propels them toward success in the data-driven era.
Categories that Drive Informed Decision-Making
Descriptive Analytics: Understanding the Past
Descriptive analytics is the foundational layer of BI analysis. It encompasses examining historical data to get insights into what happened in the past. This category provides insights into trends, patterns and key performance indicators (KPIs). Descriptive analytics answers questions like “What happened?” and “How did it happen?”
Diagnostic Analytics: Unraveling the Why
While descriptive analytics reveals what happened, diagnostic analytics delves deeper to uncover why it happened. This category involves a more in-depth analysis of historical data to identify the root causes of specific events or trends. Diagnostic analytics is concerned with understanding the factors that influenced past outcomes.
Predictive Analytics: Anticipating the Future
Predictive analytics takes a leap into the future by leveraging historical data and statistical algorithms to forecast future trends and outcomes. This category answers the question, “What is likely to happen in the future?” Predictive analytics enables proactive decisions for organizations based on anticipated scenarios.
Prescriptive Analytics: Guiding Action
Prescriptive analytics represents the pinnacle of BI analysis. Not only does it predict future outcomes but also recommends actions to optimize them. This category provides actionable insights by suggesting the best course of action to achieve desired goals. Prescriptive analytics assists in finding answers to what should be done.
Diagnostic vs. Predictive vs. Prescriptive: A Holistic Approach
While each category of BI analysis serves a specific purpose, they are not mutually exclusive. In fact, they often work together synergistically to provide a holistic view of data. The transition from diagnostic to predictive to prescriptive analytics represents a natural evolution in leveraging data for decision-making.
For instance, a manufacturing company might use diagnostic analytics to identify inefficiencies in its production process. Once the root causes are understood, predictive analytics can be employed to forecast potential future issues or bottlenecks. Finally, prescriptive analytics can recommend strategies to optimize the production process, ensuring efficiency and cost-effectiveness.
Challenges of a Successful BI Implementation
BI offers a plethora of advantages, empowering businesses to make informed decisions, optimize operations and gain a competitive edge. However, like any powerful tool, BI is not without its complexities and potential pitfalls.
Cost of Implementation and Maintenance: Implementing a comprehensive BI system involves significant upfront costs, including software, hardware and training expenses. Ongoing maintenance and updates also contribute to the total cost of ownership.
Complexity and Learning Curve: BI tools can be complex and mastering them may require a steep learning curve, particularly for non-technical users. This complexity can lead to underutilization of the tool’s capabilities and hinder its effectiveness.
Data Quality Issues: BI is only as good as the data it analyzes. If the underlying data is inaccurate, incomplete or outdated, the insights generated by BI tools may be misleading or unreliable. Maintaining data quality is an ongoing challenge for many organizations.
Integration Challenges: Integrating BI tools with existing systems and data sources can be challenging. Incompatibility issues, data silos and varying data formats may hinder seamless integration, affecting the overall effectiveness of BI initiatives.
Overemphasis on Technology: Organizations sometimes make the mistake of focusing too much on the technological aspect of BI rather than the strategic and organizational aspects. This overemphasis on technology can lead to the neglect of critical factors such as data governance, user adoption and alignment with business goals.
Resistance to Change: Introducing BI tools often requires a cultural shift within the organization. Resistance to change from employees accustomed to traditional decision-making processes can impede the successful adoption and implementation of BI initiatives.
Data Security Concerns: As BI involves the analysis of sensitive business data, security is a paramount concern. Breaches or unauthorized access to BI systems can have severe consequences, including loss of trust, legal ramifications and damage to the organization’s reputation.
Dependence on IT Support: While the goal of BI is to enable self-service analytics, users may still encounter challenges that require IT support. This dependence on IT departments can create bottlenecks and slow down the decision-making process.
Lack of Alignment with Business Goals: For BI to be effective, it must align with the strategic goals of the organization. Lack of alignment can result in irrelevant insights, wasted resources and a failure to address the specific needs of the business.
Evolving Landscape of BI Platforms
The landscape of BI platforms is continually evolving to meet the changing needs of organizations and advancements in technology. Several trends are shaping the future of BI platforms:
Integration with Artificial Intelligence (AI) and Machine Learning (ML):
The integration of AI and ML capabilities into BI platforms enhances predictive analytics, anomaly detection and automation of routine tasks. This not only improves the accuracy of insights but also enables organizations to uncover hidden patterns and trends.
Cloud-Based BI:
Cloud-based BI platforms are becoming increasingly popular due to benefits such as scalability, flexibility and cost-effectiveness. These platforms allow organizations to access BI tools without the need for extensive on-premises infrastructure, facilitating remote work and collaboration.
Natural Language Processing (NLP):
NLP capabilities are being integrated into BI platforms to enable users to interact with data using natural language queries. This makes BI tools more accessible to non-technical users, democratizing data across the organization.
Data Storytelling:
BI platforms are increasingly focusing on enhancing data storytelling capabilities. This involves presenting data in a narrative format, making it easier for users to understand the context and implications of the insights derived from the data.
Augmented Analytics:
Augmented analytics leverages AI and ML to automate insights generation. It assists users in identifying relevant patterns and trends in the data, reducing the manual effort required for analysis.
Real-Time Analytics:
The demand for real-time analytics is growing as organizations seek to make decisions based on the most up-to-date information. BI platforms are incorporating features that enable real-time data processing and analysis.
Understanding Big Data
Big Data refers to massive volumes of structured and unstructured data that inundate businesses on a day-to-day basis. This data comes from a myriad of sources, including social media, sensors, transaction logs, and more. The key characteristics of Big Data are often summarized using the three Vs: Volume, Velocity, and Variety.
Volume: Big Data involves vast amounts of information. Traditional databases struggle to handle the sheer volume of data generated by modern businesses.
Velocity: Data is generated at an unprecedented speed. Real-time data processing has become crucial for businesses to react swiftly to changing circumstances.
Variety: Data may come in varying formats, namely structured, semi-structured and unstructured. These can range from text, images and videos to social media posts. Managing this diverse range of data types is a challenge.
While Big Data provides the raw material, business intelligence acts as the refining process, turning this raw data into actionable insights. BI is the strategic approach and technology-driven process that businesses employ to analyze data and make informed decisions. The integration of BI and Big Data creates a powerful synergy that empowers businesses to extract maximum value from their data.
In conclusion, the world of business intelligence is a dynamic and transformative landscape that empowers organizations to make data-driven decisions and stay competitive in today’s fast-paced business environment. As businesses continue to amass vast amounts of data, the role of BI becomes increasingly crucial in extracting valuable insights. Embracing business intelligence not only enhances decision-making processes but also fosters a culture of continuous improvement and innovation. In this era of information, the ability to harness and leverage data strategically is a key differentiator and business intelligence serves as the guiding light for organizations aiming to navigate the complexities of the modern business world.