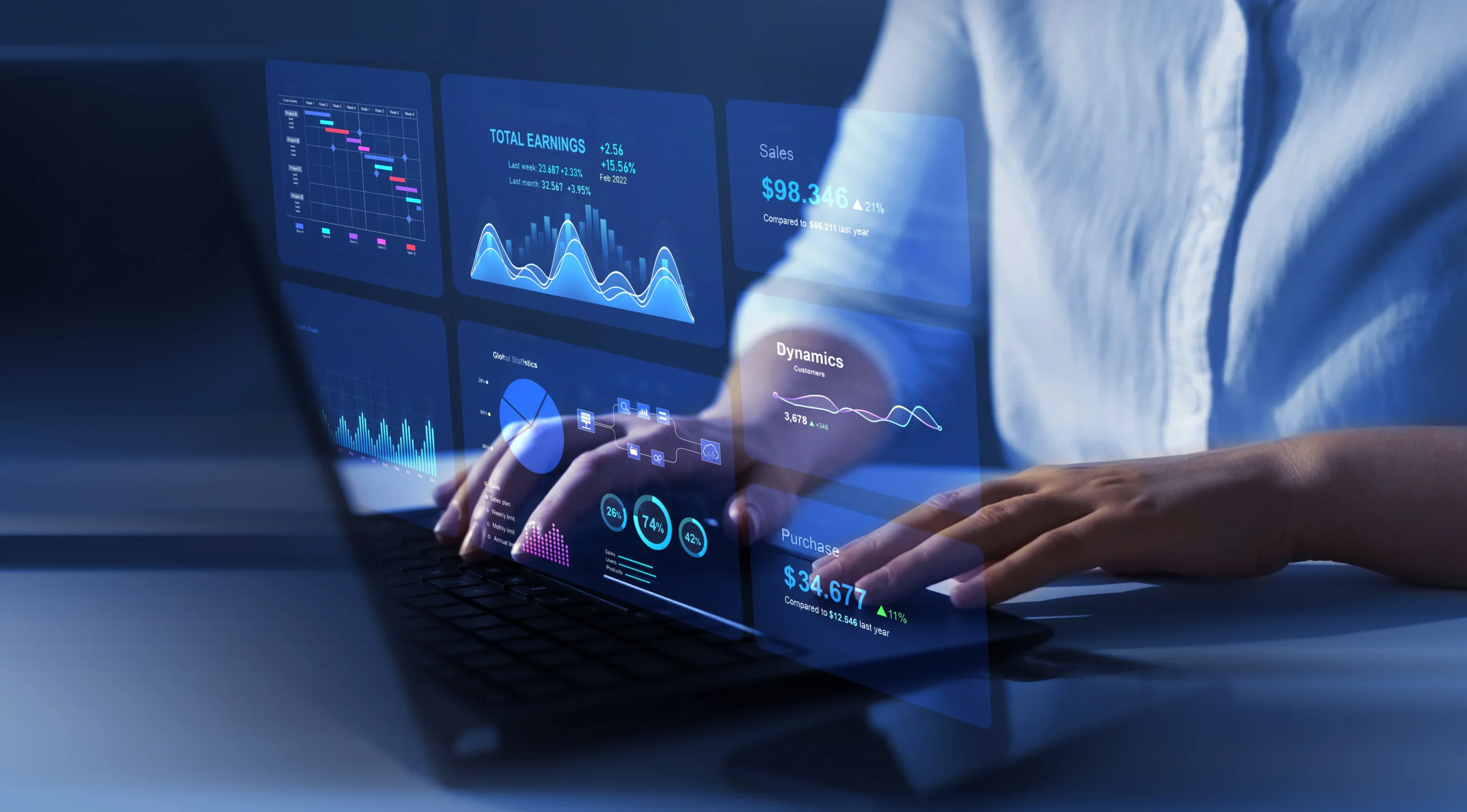
Business Analytics: A Comprehensive Guide
Businesses of all sizes, from small startups to large corporations in various industries, recognize the potential of data and analytics in driving growth and digital transformation.
By combining these insights, business-focused analytics provides valuable perspectives on business performance trends. This comprehensive guide will explore the realm of business analytics, its importance, fundamental concepts, real-world applications and much more.
What Is Business Analytics and Why Is It Important?
Business analytics is the process of analyzing and interpreting data to gain valuable insights into business performance and identify areas for improvement. This can be done using various techniques and tools, such as predictive analytics, natural language processing and machine learning to extract meaningful information from structured and unstructured data sources.
Business-focused analytics is applicable to various industries and job functions. Regardless of the specific context, the main objective remains consistent, i.e., to discover patterns, trends, correlations and other valuable information that can guide decision-making processes, improve operations and support strategic planning efforts.
By adopting a data-driven approach through advanced analytics, organizations can make well-informed decisions based on factual evidence rather than relying solely on intuition or instinct. By unveiling hidden patterns and trends, they can drive innovation, enhance customer satisfaction and provide a competitive advantage.
Components of Business Analytics
To extract value from data, Business-focused Analytics consists of several essential components:
Data Mining
By applying techniques such as machine learning, statistical analysis and database querying, valuable insights are revealed to uncover hidden patterns, trends and correlations within extensive datasets. For example, businesses can employ data mining to understand influential factors behind customer purchasing decisions or identify impactful marketing strategies.
Text Mining
Text mining focuses on extracting meaningful information from unstructured text data. Through the application of natural language processing, businesses can analyze various textual sources such as customer reviews, social media posts, emails and more. This enables them to gauge customer sentiments, understand opinions and identify emerging trends. By understanding customer feedback, businesses can refine their products, services and marketing approaches.
Data Aggregation
Data aggregation is the collection and summarization of data from different sources, making this information accessible and understandable for analysis. By bringing together data from disparate systems, departments or platforms, businesses can gain a comprehensive view of their operations. This enables decision-makers to evaluate overall performance, identify areas of improvement and explore opportunities for growth.
Forecasting
Forecasting uses historical data and statistical models to predict future trends and outcomes. By analyzing patterns, businesses can anticipate changes in demand, market trends or customer behavior to effectively plan resources, manage inventory and allocate budgets. For example, retailers often utilize forecasting to optimize stock levels for upcoming seasons.
Data Visualization
Data visualization offers the skillful presentation of complex data in a visual format, facilitating comprehension and analysis. Through charts, graphs, heatmaps and interactive dashboards, raw data is transformed into captivating visuals. This empowers decision-makers to swiftly grasp insights and detect trends or outliers, ultimately enabling them to make well-informed choices, especially when sharing valuable information with non-technical stakeholders.
Types of Business Analytics
Here’s an overview of the different types of business-oriented analytics:
Descriptive Analytics
By gathering and analyzing historical data, descriptive analytics helps gain a comprehensive understanding of past events within a business. This analytical approach empowers organizations to generate summaries, reports and visualizations that highlight trends, patterns and key performance indicators (KPIs). Descriptive analytics lays the groundwork for advanced analytical techniques by providing decision-makers with a clear comprehension of their business’s historical context.
Diagnostic Analytics
Diagnostic analytics goes beyond descriptive analytics by providing answers to the “why” behind trends and patterns. It explores causality, uncovering the underlying reasons. This type of analysis delves into correlations and relationships within data, allowing businesses to identify the contributing factors to specific outcomes.
For instance, when sales increase during a specific period, diagnostic analytics can determine whether marketing campaigns, product launches or external events are responsible for the growth. Such analysis is essential for recognizing improvement opportunities and making strategic adjustments based on a deeper understanding of past performance.
Predictive Analytics
Looking ahead into the future with predictive analytics, organizations utilize historical data and advanced algorithms to predict forthcoming trends, behaviors and events. This analysis uncovers patterns in past data that can be leveraged for predictions about future occurrences. For example, predictive analytics aids businesses in anticipating customer preferences, navigating demand fluctuations and staying updated on market trends. By proactively identifying potential outcomes, organizations can strategically adjust their approaches, allocate resources more effectively and position themselves to seize upcoming opportunities.
Prescriptive Analytics
Prescriptive analytics takes data analysis to a proactive level. It suggests optimal actions for achieving desired outcomes, combining historical data, predictive models and business rules. By recommending specific steps that lead to the best results, prescriptive analytics enables businesses to maximize profitability within budget constraints. From pricing strategies to marketing campaigns and supply chain adjustments as potential solutions, decision-makers can leverage these insights to align their choices with overall business objectives.
The Business Analytics Process
The process of business analytics follows a structured path, converting raw data into actionable insights that help organizations make informed decisions and achieve positive outcomes. These steps comprise various key stages, each playing an important role in extracting valuable information from the data. Now let’s delve into these steps and explore them further:
Define the Problem
The process starts by identifying the business problem or question that requires attention. This can include enhancing customer retention or optimizing supply chain operations. Defining a clear problem statement sets the course for the analysis and ensures that the insights align with the organization’s goals.
Data Collection
Once the problem is identified, data is collected from various sources to address it. These sources can range from internal databases like transaction records, customer information, etc., to external channels like surveys and social media. The accuracy of the analysis relies on both the quality and quantity of the gathered data.
Data Cleaning and Preparation
Raw data often contains errors, inconsistencies and missing values. During the data cleaning stage, the information is refined and converted into a format that can be effectively utilized. This process encompasses tasks like eliminating duplicates, addressing missing values and ensuring overall data coherence. Additionally, preparing the data may involve structuring it in a manner conducive to analysis, such as tables or databases.
Exploratory Data Analysis (EDA)
By visually and statistically exploring the dataset to uncover initial insights, this step aids analysts in comprehending data distributions, correlations, outliers and potential patterns. To gain a deeper understanding of the data, they can employ visualization tools and techniques to create charts, graphs and summaries.
Data Modeling and Analysis
During this phase, analysts apply statistical models, algorithms and machine learning to the prepared data. They use various methods such as regression analysis, clustering, classification or time series forecasting based on the specific problem. The ultimate objective is to extract meaningful patterns, relationships and trends that offer valuable insights into business problems.
Interpretation of Results
The analysis results are interpreted within the framework of the initial problem statement. Analysts address the questions raised earlier and provide insights that tackle the business challenge at hand. This crucial step involves transforming complex analytical findings into practical recommendations through visual aids that businesses can quickly grasp.
Monitoring and Feedback
Continuous monitoring entails tracking relevant metrics, analyzing ongoing data and comparing results against expected outcomes. The feedback gathered from these strategies contributes to refining the approach to analytics processes.
Benefits of Business Analytics
Business-oriented analytics offer many benefits that contribute to the growth and success of organizations across various industries. Here are some key advantages:
Examples of Business Analytics
The following are some real-world examples that illustrate how businesses from various industries and functional areas have effectively utilized business analytics.
Business analytics uses advanced statistical analysis and predictive modeling to support proactive decision-making and forecast future trends.
Business intelligence is a practice that involves analyzing historical and current data to gain insights about past events and performance. This analysis is done using tools such as reporting and data visualization.
Data analytics is a field that uses techniques to extract valuable insights from raw data. It involves processes like data cleaning, transformation and statistical analysis. This field finds applications in various domains, such as scientific research and healthcare.
Here’s a table summarizing their differences:
Aspect | Business Analytics | Business Intelligence | Data Analytics |
Focus | Future-oriented: Predicting trends, outcomes and making informed decisions. | Past and Present: Analyzing historical data to support strategic decision-making. | Present: Analyzing current data for insights and patterns. |
Objective | Drive future actions and outcomes by providing insights for proactive decisions. | Support decision-making through historical data analysis and reporting. | Uncover insights and trends within existing datasets. |
Techniques | Advanced statistical analysis, predictive modeling, machine learning, data mining. | Reporting, querying, data visualization tools, OLAP (Online Analytical Processing). | Statistical analysis, data cleaning, transformation, data exploration. |
Scope | Strategic planning and operational optimization across various business functions. | Monitoring KPIs, identifying trends and understanding historical performance. | Application across diverse domains, including social sciences, healthcare, and finance along with business functions |
Best Practices for Business Analytics
By adhering to the following practices, businesses can effectively leverage insights to enhance operations and decision-making processes.
Defining Clear Business Use Cases and Goals
In the beginning of their analytics journey, organizations need to define the business problems they aim to solve and the objectives they want to achieve. By collaborating with stakeholders from different departments, they can identify areas of concern and potential growth opportunities. This collaborative effort ensures that analytics align with the overall corporate strategy.
Setting Well-Defined Success Criteria
The success of analytics relies heavily on clear and measurable metrics. By crafting quantifiable criteria directly tied to the goals identified, businesses can effectively evaluate how analytics impact their overall performance. These metrics act as benchmarks for assessing the effectiveness of enhancements driven by analytics.
Validating Models with Success Criteria
Organizations should conduct rigorous testing to assess the developed models against predefined success and failure criteria. This validation process serves as a safeguard, guaranteeing the accuracy and dependability of models before their deployment, ultimately enhancing the quality of generated insights.
Developing a Comprehensive Methodology
A structured methodology involves carefully curating data, considering internal and external factors that influence outcomes. By understanding the variables contributing to results, organizations can create accurate predictive models. Additionally, incorporating an agile approach and utilizing project management tools help streamline the implementation of these models and make iterative improvements.
Challenges of Business Analytics
There are several challenges faced on a business analytics journey, including:
Intellicus offers a comprehensive suite of solutions in the field of business analytics, addressing the various obstacles that organizations commonly face. Here’s how:
Streamlining IT Involvement and Infrastructure
To minimize IT involvement, businesses can leverage Intellicus’ high-speed OLAP dashboards and real-time analytics. The platform helps promote collaboration between business users and the IT department, encouraging independent data access and analysis to reduce conflicts. It is also crucial to establish clear communication channels, define roles, and develop a shared vision using Intellicus as a tool for facilitating a cohesive approach between IT and business units.
Simplifying Complexity of Analytical Techniques
Intellicus efficiently addresses the complexities of choosing the appropriate analytical techniques head-on. By leveraging its built-in data science and machine learning capabilities, Intellicus empowers users to proactively envision future possibilities and make well-informed decisions. The support for popular data science engines like R and Python adds flexibility, allowing users to select from a range of preloaded scripts that streamline their analytics processes.
Maximizing ROI And Overcoming Cost Challenges
Intellicus empowers organizations to optimize their return on investment (ROI) through a comprehensive platform that caters to diverse business needs. With self-service ad hoc reporting and real-time analytics, they can efficiently derive valuable insights. The intuitive report builder allows customization according to specific business requirements while integrated AI capabilities enhance the accuracy of predictive analysis.
Future of Business Analytics
The integration of AI and machine learning will enhance the accuracy of predictive and prescriptive analytics, making them even more powerful.
Moreover, the availability of diverse data sources, including IoT devices, will provide a wealth of information for analysis. Streamlined processes through automated data preparation and cleaning will maximize efficiency. Real-time analytics will empower businesses to respond to market changes swiftly.
Additionally, ethical data usage and responsible AI practices are vital considerations in this field. As the demand for data-driven insights increases, professionals skilled in business analytics will have an essential role in shaping business strategies and driving innovation across industries.
Conclusion
Business analytics involves strategically transforming data into valuable insights that drive innovation and strategic decision-making. By analyzing patterns, trends and correlations, organizations gain a competitive advantage, enhance operations and improve customer experiences. Advancements in AI and real-time analytics are poised to revolutionize its potential even further.